-
-
Notifications
You must be signed in to change notification settings - Fork 25.9k
EHN Add transform_inverse to Nystroem #19971
New issue
Have a question about this project? Sign up for a free GitHub account to open an issue and contact its maintainers and the community.
By clicking “Sign up for GitHub”, you agree to our terms of service and privacy statement. We’ll occasionally send you account related emails.
Already on GitHub? Sign in to your account
Draft
kstoneriv3
wants to merge
10
commits into
scikit-learn:main
Choose a base branch
from
kstoneriv3:feature/inverse_transform_nystroem
base: main
Could not load branches
Branch not found: {{ refName }}
Loading
Could not load tags
Nothing to show
Loading
Are you sure you want to change the base?
Some commits from the old base branch may be removed from the timeline,
and old review comments may become outdated.
Draft
EHN Add transform_inverse to Nystroem #19971
kstoneriv3
wants to merge
10
commits into
scikit-learn:main
from
kstoneriv3:feature/inverse_transform_nystroem
Conversation
This file contains hidden or bidirectional Unicode text that may be interpreted or compiled differently than what appears below. To review, open the file in an editor that reveals hidden Unicode characters.
Learn more about bidirectional Unicode characters
kstoneriv3
commented
Apr 24, 2021
kstoneriv3
commented
Apr 24, 2021
sklearn/kernel_approximation.py
Outdated
@@ -778,11 +836,17 @@ def fit(self, X, y=None): | ||
**self._get_kernel_params()) | ||
|
||
# sqrt of kernel matrix on basis vectors | ||
U, S, V = svd(basis_kernel) | ||
U, S, V = svd(basis_kernel) # TODO(kstoneriv3): Why not np.linalg.eigh() ? |
There was a problem hiding this comment.
Choose a reason for hiding this comment
The reason will be displayed to describe this comment to others. Learn more.
I suppose eigh
is faster than svd
as the former is tuned specifically for Hermitian matrices. There is a comparison in the page below.
https://stackoverflow.com/questions/50358310/how-does-numpy-linalg-eigh-vs-numpy-linalg-svd
cc @ogrisel @lorentzenchr maybe? |
Sign up for free
to join this conversation on GitHub.
Already have an account?
Sign in to comment
Add this suggestion to a batch that can be applied as a single commit.
This suggestion is invalid because no changes were made to the code.
Suggestions cannot be applied while the pull request is closed.
Suggestions cannot be applied while viewing a subset of changes.
Only one suggestion per line can be applied in a batch.
Add this suggestion to a batch that can be applied as a single commit.
Applying suggestions on deleted lines is not supported.
You must change the existing code in this line in order to create a valid suggestion.
Outdated suggestions cannot be applied.
This suggestion has been applied or marked resolved.
Suggestions cannot be applied from pending reviews.
Suggestions cannot be applied on multi-line comments.
Suggestions cannot be applied while the pull request is queued to merge.
Suggestion cannot be applied right now. Please check back later.
Reference Issues/PRs
As discussed in
#19732#19899,sklearn.kernel_approximation.Nystroem
currently lackstransform_inverse()
method unlikePCA
andKernelPCA
.What does this implement/fix? Explain your changes.
This PR implements
transform_inverse
insklearn.kernel_approximation.Nystroem
.Any other comments?
While implementing the method, I was not confident about the choice of linear system solver APIs. Maybe there is a better API for solving systems with a positive semidefinite matrix.
The formula I used for kernel ridge regression of original data points to the low-dimensional representation vectors are as follows (it is also commented in the code).

The reconstruction performance was compared with KernelPCA using the example in #19945. The reconstruction quality seems identical in this case.
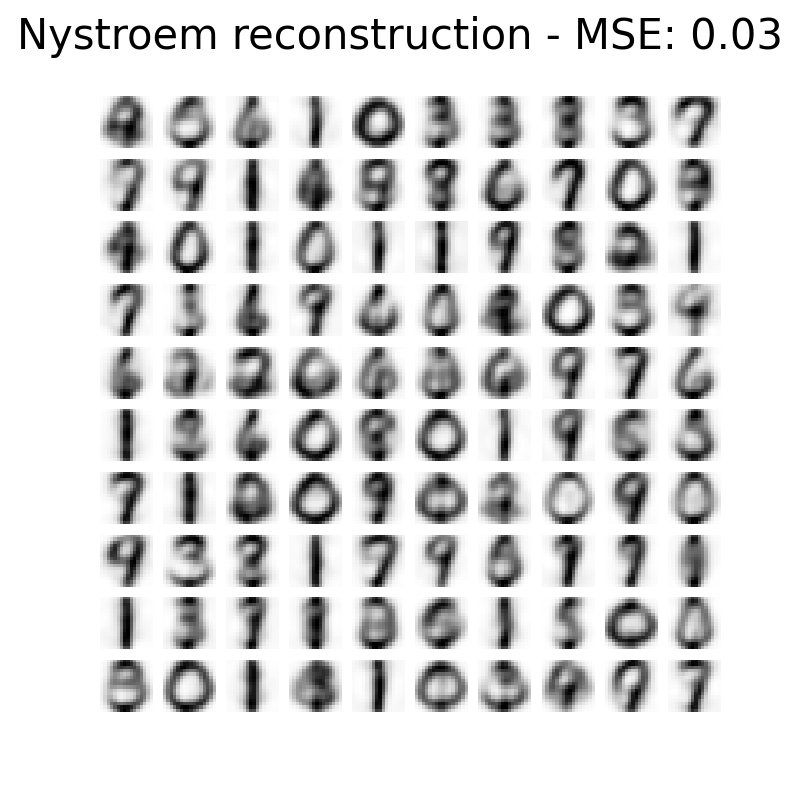
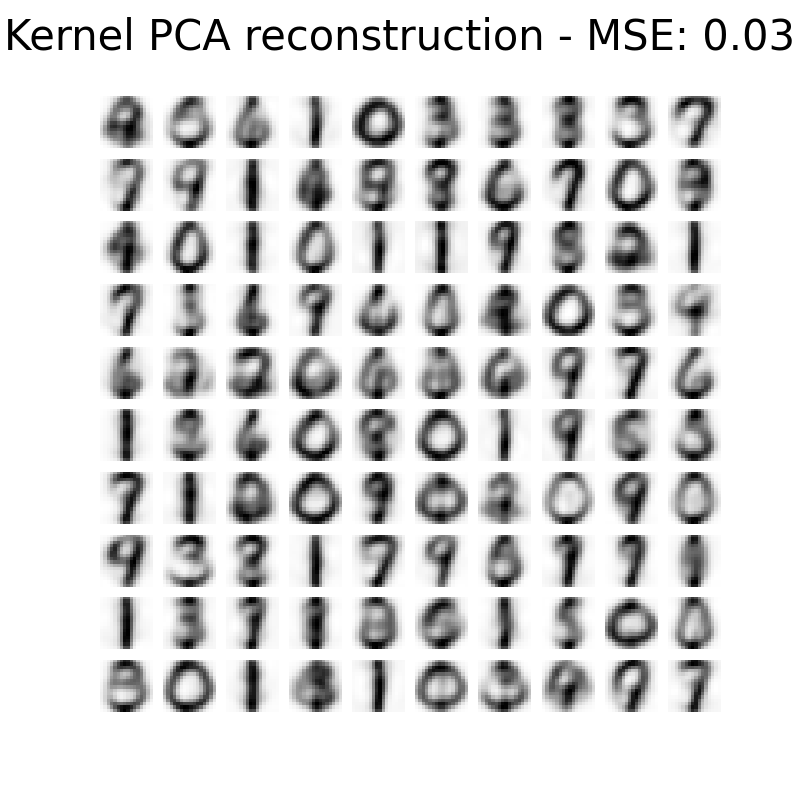